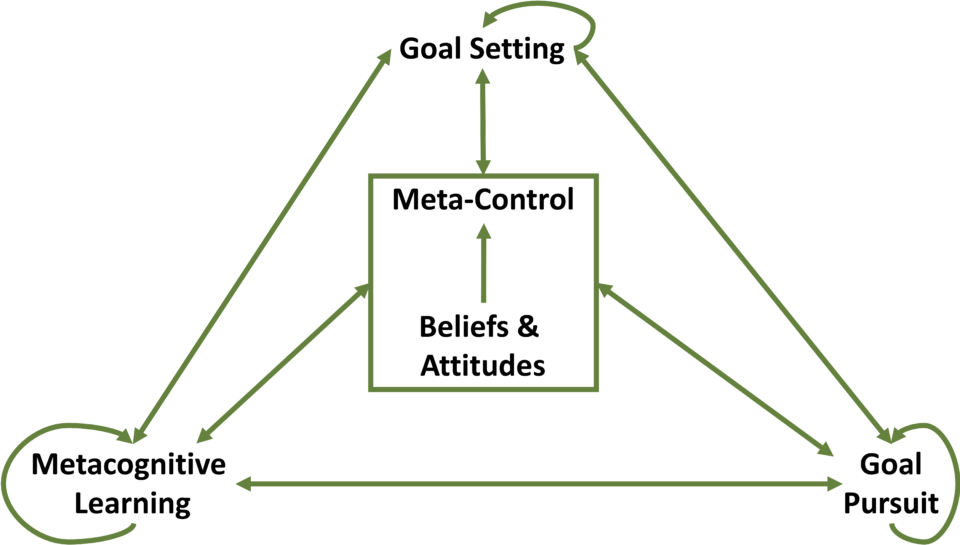
Our research on the computational principles of human intelligence strives to elucidate and reverse-engineer two of the most impressive features of the human mind: proactivity and metacognitive learning. To reverse-engineer the underlying computational mechanisms we have developed a new cognitive modeling paradigm called resource-rational analysis that has been rapidly adopted by many cognitive scientists.
Resource rationality and rational metareasoning
Our most influential scientific contribution so far has been to develop and establish the theory of resource rationality as a framework for modeling the computational mechanisms of human intelligence. This new approach to cognitive modeling integrates the functional constraints imposed by the goal of a cognitive mechanism with the computational constraints imposed by people’s finite time and bounded cognitive resources. This new approach to cognitive modeling has spread rapidly, with the 16 articles in which we introduced and established it being collectively cited more than 1400 times in the first six years.
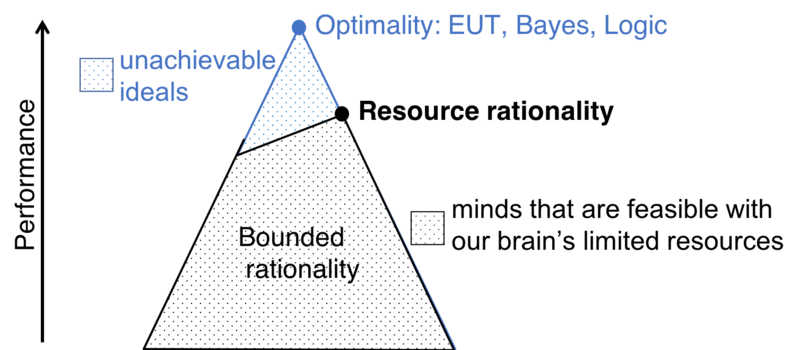
One of our recent theoretical contributions has been to develop and test computational models of two important metacognitive abilities that contribute to people’s resource-rationality, namely the adaptive control of reasoning and decision-making and metacognitive learning (see below; \footfullcite{HeJainLieder2021NIPS-Planning)). The progress summarized here and in other sections of this report demonstrates that the Rationality Enhancement Group continues to successfully leverage the theory of resource-rationality to reverse-engineer, augment, and enhance human cognition.
Proactivity
An essential aspect of human intelligence is that people often take the initiative to set and pursue their own goals instead of merely reacting to their environment. This is known as proactivity. To elucidate the computational principles of proactivity, the Rationality Enhancement Group has developed resource-rational models of how people pursue their goals, new methods for elucidating the cognitive strategies of human planning, and a rational metareasoning model of how the brain decides when to engage the mechanisms of proactivity. To facilitate research on human planning we developed a Bayesian method for inferring people’s mental planning strategies from their overt behavior in an appropriately designed planning task. This led us to discover that people make adaptive use of 79 planning strategies of 13 different types. In addition, we have developed a machine learning method for clustering and describing people’s planning strategies automatically.
Metacognitive Learning
How does the human brain learn how to think and how to decide? What are the learning mechanisms that give rise to human intelligence and enable us to get better at what we do? How can this learning be promoted and accelerated? To answer these questions, we reverse-engineer how people learn when to use which cognitive strategy (Lieder, Plunkett, et al., 2014; Lieder & Griffiths, 2017;), how the brain learns to control its own information processing, and how people discover and continuously refine their own cognitive strategies. To make this possible, we have developed new empirical and computational methods for measuring learning-induced changes in people’s planning strategies. We have used these methods to characterize metacognitive learning empirically and our models of metacognitive learning can capture not only how people’s average performance improves with practice but can also predict the underlying qualitative changes in people’s planning strategies. So far, this line of research has led to 9 publications and 399 citations.
In a follow-up project, we are currently investigating whether reflection can improve metacognitive learning and what the underlying mechanisms might be. The resulting psychological insights and technical advances lay a scientific foundation for leveraging technology to accelerate human learning and improve human decision-making. Our initial results show that guiding people to systematically reflect on how they make their decisions by asking them a series of Socratic questions leads to instantaneous metacognitive learning.